Abstract
Metagenomic approaches are now commonly used in microbial ecology to study microbial communities in more detail, including many strains that cannot be cultivated in the laboratory. Bioinformatic analyses make it possible to mine huge metagenomic datasets and discover general patterns that govern microbial ecosystems. However, the findings of typical metagenomic and bioinformatic analyses still do not completely describe the ecology and evolution of microbes in their environments. Most analyses still depend on straightforward sequence similarity searches against reference databases. We herein review the current state of metagenomics and bioinformatics in microbial ecology and discuss future directions for the field. New techniques will allow us to go beyond routine analyses and broaden our knowledge of microbial ecosystems. We need to enrich reference databases, promote platforms that enable meta- or comprehensive analyses of diverse metagenomic datasets, devise methods that utilize long-read sequence information, and develop more powerful bioinformatic methods to analyze data from diverse perspectives.
Data deluge in microbial ecology
Although microbes play fundamental roles in various ecosystems, most have not yet been characterized in detail. Bioinformatics, which aims to discover new biological concepts and laws based on large-scale data, is now expected to accelerate discovery in unexamined areas of the microbial universe. The data deluge has made bioinformatics indispensable in modern research; recent innovative technologies are producing large amounts of data at an unprecedented pace. Observations are key to science; for example, optical and electron microscopies are important methods of observation combined with various staining methods. Among recent observational technologies, high-throughput DNA sequencing technologies have rapidly produced vast amounts of genetic information at low cost, making available thousands of microbial genomes. These genome sequences provide a comprehensive catalog of the microbial genetic elements underlying diverse microbial physiology, and also assist in weaving a massive tapestry of microbial evolutionary histories (72, 154).
In microbial ecology, research has been hindered because the majority of environmental microbes are uncultivable. A large number of studies across diverse natural environments have identified many microbial groups with no axenic culture (110, 113, 133, 138). In order to overcome this fundamental difficulty, culture-independent approaches, including DNA hybridization (e.g., microarray and fluorescent in situ hybridization), DNA cloning, and PCR have been used to detect specific members and/or functional genes in microbial communities (3, 8, 9, 32, 34, 47, 56, 61, 74, 157, 174, 185). High-throughput sequencing technologies have recently popularized shotgun metagenomic and (typically 16S ribosomal RNA [rRNA] gene) amplicon sequencing methods, which identify members and/or functional genes at a greater scale and in more detail. Their use in diverse environments has revealed the presence of extremophiles (27, 33, 92), uncovered relationships between microbes and human diseases (10, 44, 55, 57, 85, 87, 96, 134, 147), and characterized the nutrition systems involved in symbiosis (68, 174, 177). Even more applications of these methods are used in agriculture (93), food science and pharmaceuticals (32), and forensics (49, 79, 82, 182). Many large-scale metagenomic projects are now generating comprehensive microbial sequence collections for different environments (e.g., human-associated [116, 167], soil [54, 171], and ocean environments [17, 142]). Since microbial communities change as they interact with other organisms and as the environment changes, time-series analyses have also become common (21, 24, 77, 115, 172).
Several bioinformatic tools have been developed and popularized to analyze metagenomic and amplicon sequence data. Web servers, such as MG-RAST (104), IMG/M (97), EBI Metagenomics (69), and SILVAngs (135), and pipelines, such as MEGAN (70), QIIME (25), and Mothur (145), now allow researchers to perform integrated metagenomic analyses and visualize results without command-line operations or strong computational knowledge. Since there are already several introductory articles on these popular tools (36, 88, 103, 123, 146), we herein addressed how can we examine large datasets in detail in order to obtain a deeper understanding of the ecology and evolution of microbes in the environment beyond existing approaches that are already popular (Fig. 1).
Toward better taxonomic assignments
A fundamental step in microbial ecology is to describe the taxonomic distribution of microbial community members. Thus, the precise taxonomic assignment of sequencing reads is one of the most important issues in the analysis of metagenomic and amplicon sequencing data. Reference-based methods are frequently used for this purpose, in which taxonomic assignments are based on straightforward sequence similarity searches against reference genomes (e.g., RefSeq [163]) or 16S rRNA sequence databases (e.g., Greengenes [101], SILVA [135], RDP [31], and Ez-Taxon [29]). These databases typically contain the sequences of previously isolated and taxonomically classified strains, whereas they also contain environmental clone sequences. Many bioinformatic tools, such as TANGO (6), MetaPhlAn (148), and Kraken (176), have been developed to improve the computational efficiency, accuracy, and sensitivity of taxonomic assignments. Although these tools perform well for many applications, discriminating between closely related species is sometimes difficult, particularly in cases of highly conserved genes (e.g., 16S rRNA genes). Additionally, genes that undergo horizontal gene transfer (HGT) between different taxa may cause incorrect taxonomic assignments. A more fundamental issue is taxonomic bias in reference databases, which leads to biased taxonomic assignments. A previous study reported that taxonomic assignments markedly change when different versions of reference databases are used (128). Therefore, even in this era of data deluge, the further taxonomic enrichment of reference databases is key to the improvement of reference-based methods. It is important to note that this issue is more crucial in the analysis of fungal and viral sequences because fewer reference sequences are available and their taxonomy is under debate. In order to overcome this obstacle, several projects are now attempting to obtain a number of genomic sequences to enrich databases (58, 180). In cases in which amplicon sequencing data are analyzed, the filtering of chimeric sequences formed during PCR is very important for precise analyses (63). Several bioinformatic tools, such as AmpliconNoise (136), ChimeraSlayer (63), and UCHIME (41), have been proposed and commonly used to remove chimeric sequences.
Reference-free methods may be used (e.g., CD-HIT [50], UCLUST [40], and UPARSE [42]) as an alternative to reference-based methods. These methods use clustering to group marker genes, such as 16S rRNA, ribulose-1,5-bisphosphate carboxylase/oxygenase (RuBisCO), ammonia monooxygenase (amoA), sulfate thioesterase/thiohydrolase (soxB), and methyl-coenzyme M reductase genes (mcrA), into unique representative sequences that serve as operational taxonomic units (OTUs) (27). 16S rRNA genes are used to study the general composition of a microbial community, while RuBisCO, amoA, soxB, and mcrA genes are typically used to investigate microbes that play critical roles in carbon, nitrogen, sulfur, and methane cycles, respectively. In addition to traditional genes, useful marker genes may be found and used by comprehensively profiling metagenomic datasets (159). In reference-free methods, OTUs often cannot be assigned to known taxa. In order to estimate phylogenetic information for these OTUs, PhylOTU (150), pplacer (98), and PhyloSift (35) couple reference-free methods with phylogenetic analyses.
Toward the cultivation-free reconstruction of genomic sequences
Most metagenomic studies currently focus at the level of individual genes (“gene-centric” metagenomics [132]). In contrast, in some pioneering research on “genome-centric” metagenomics, microbial genomes that include those of important uncultivated taxonomic groups were successfully reconstructed by metagenomic binning and assembly from various environments including oceans, groundwater, soil, hypersaline lakes, and acid mine drainage (4, 18, 45, 95, 112, 168). Although amplification bias still poses a non-negligible difficulty, single-cell genomic sequencing is expected to accelerate direct genome reconstruction from environmental samples (43, 90, 140), in which the combination of single cell genomic and metagenomic approaches may be a promising approach (102).
Metagenomic assembly is an important step for revealing the ecology and physiology of environmental microbes, in which the fundamental concepts of metagenomic assembly from short-read sequences have already been described in detail (36, 88, 103, 123, 146). Several tools have been developed for metagenomic assembly, and are classified into reference-based (e.g., AMOS [130]) and de novo methods (e.g., MetaVelvet-SL [2], SPAdes [119], and IDBA-UD [127]). In the case of de novo assembly, users need to consider chimeric contigs because similar genetic regions may be shared by different genomes (100, 129, 170). In order to improve the performance of de novo metagenomic assembly, composition-based methods use specific sequence features in a metagenomic dataset to split reads into different species. For example, CONCOCT (5), metaBAT (81), and MaxBin (178) bin sequences based on their tetra-nucleotide frequency composition and coverages. These composition-based approaches are computationally intensive, particularly in their memory usage. Thus, a fast-clustering approach using matrix decomposition with streaming singular value decomposition may be combined (30). On the other hand, sequence coverage information across different DNA extraction methods may also be used to effectively split sequences into species because the numbers of sequence reads from the same genome need to be similar regardless of the extraction method (4). A related approach bins co-abundant sequences across a series of metagenomic samples from similar environments (e.g., human gut microbiome) to identify co-abundance gene groups (117).
Another information source that may improve the performance of metagenomic assembly is long-range contiguity. The recent development of methods to investigate long-range chromatin interactions (e.g., Carbon-Copy Chromosome Conformation Capture [5C] [38] and Hi-C [11]) may also contribute to metagenomic assembly because these methods ligate sequences from two different genomic regions that are in the same cell (20). The Irys system (BioNano Genomics, San Diego, USA), which also detects long-range contiguity with fluorescently labeled DNA, may be used to obtain long contigs (64).
Toward a more reliable estimation of community metabolism
Microbial genomes are affected by the environment during their evolution. Metabolic processes encoded in the genome, from biosynthesis to biodegradation, directly link microbial communities to the environment. Since most microbes are uncultivable, the direct estimation of community-scale metabolic pathways is also targeted by a metagenomic analysis. The most straightforward approach is to conduct sequence-similarity searches against pathway databases, such as KEGG (80), MetaCyc (22), and SEED (124), and use the findings obtained to annotate metabolic genes. Since many pathways with component genes that are only partially found in given metagenome data are typically detected with this naïve approach, MAPLE (160), MinPath (183), MetaNetSam (75), and HUMAnN (1) quantitatively or probabilistically evaluate whether these pathways likely function, enabling comparisons between samples. Significant biases in the databases of known pathways need to be taken into consideration when interpreting the findings of these methods. If shotgun metagenome data are unavailable, “virtual metagenomes” or functional gene abundance may be estimated using 16S rRNA amplicon sequencing data (89, 121). This approach takes advantage of closely related genomes being more likely to have a similar gene content, and, thus, given the 16S rRNA sequence, the gene content of its host genome may be estimated (at least, to some extent) if a closely related genome is already sequenced. It is important to note that such estimations may become difficult when applied to microbial groups with genomes that are rarely available and also that genomic variations within closely related microbial groups cannot be precisely considered. Despite these difficulties, this approach is very cost-effective and more easily applicable to large-scale comparative analyses.
Toward a community-level analysis of genomic structural variations and dynamics
Operon structures, which are unique to prokaryotic genomes, reflect the function of their encoded genes and need to be associated with microbial ecological strategies. Thus, if we observe systematic variations in the gene order (or gene cluster structures) due to gene losses, fusions, duplications, inversions, translocations, and HGTs from an analysis of metagenome data, these variations may provide important clues for linking microbial communities to the environment (Fig. 2A). Although difficulties are sometimes associated with distinguishing variations under selection pressure from those because of population changes, MaryGold (118) is a tool for the visual inspection of such variations. Variations in the gene order for genes in the tryptophan pathway were identified within contigs assembled from the Sargasso Sea metagenome (78). Since the availability of long sequences that encompass multiple genes greatly facilitates a gene-order analysis, DNA cloning may also be used if the targeted pathways are efficiently enriched by colony selection (51, 158). On a larger scale, gene order may be affected by genome replication mechanisms. Most prokaryotic genomes are circular with one replication origin; thus, genes close to the origin may physically exist in multiple copies, particularly during an active growth phase. Thus, the detection of these regions from metagenomic sequences may reveal the growth dynamics of microbes in a community (86).
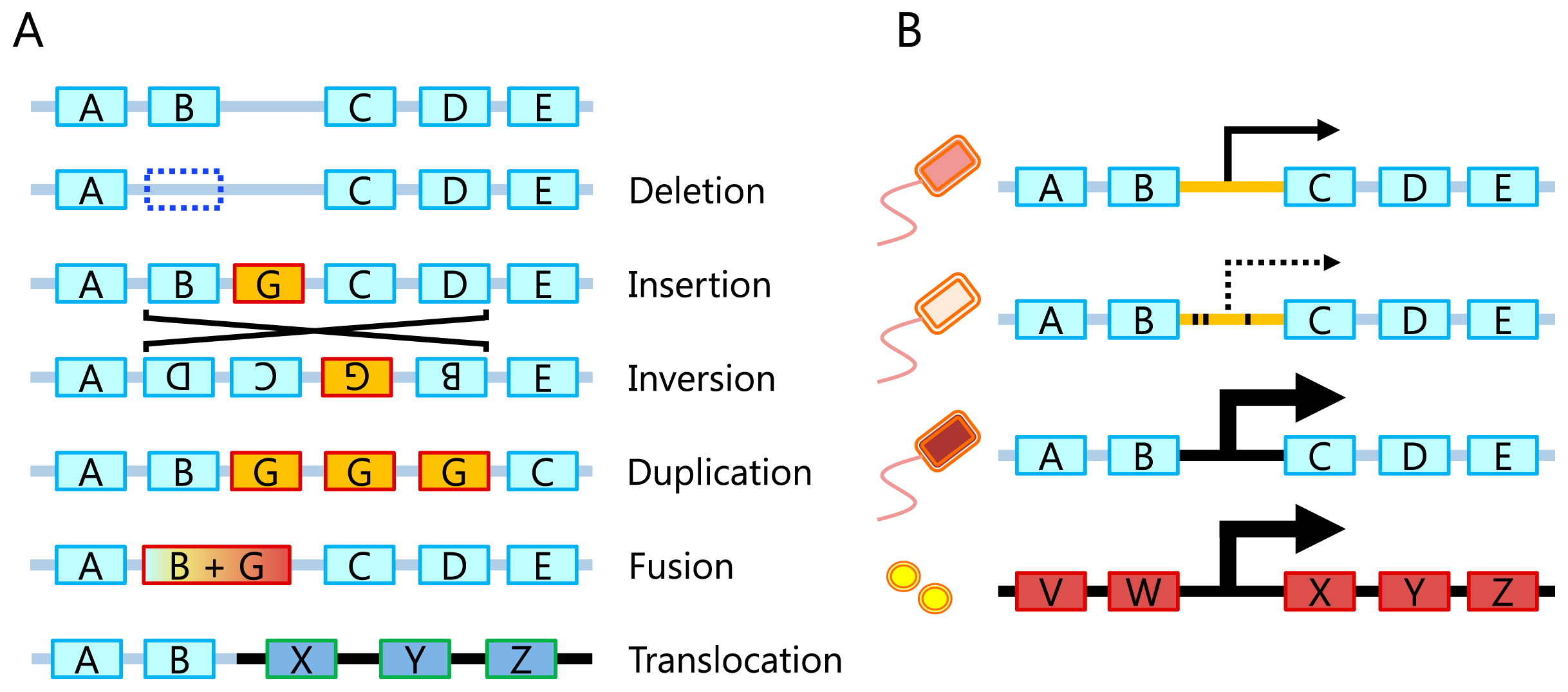
Among various sources of genomic variations and dynamics, HGT is of particular interest because it may help microbes adapt quickly to different environments (120, 139, 156). Although many comparative genomic studies have analyzed HGT (26, 73, 153), its role in microbial communities has not yet been elucidated in detail. A classical approach to detect HGT within communities is based on DNA cloning, which is particularly effective if colony selection is applied to the targeted genes (162, 175). In metagenomics, assembled contigs may be used to comprehensively identify HGT events by analyzing phylogenetic incongruence and gene order differences (62, 125, 161). In addition, gene gains via plasmids are also important driving forces that accelerate microbial adaptation to their environment. In accordance with this hypothesis, plasmids are frequently reported to contain genes that may contribute to fitness, such as detoxification genes (151, 152, 165) and antibiotic resistance genes (13). Notably, plasmid-specific metagenomics, or a plasmidome analysis, is now being conducted to directly investigate environmental plasmids without culturing or cloning (37, 173). For example, the bovine rumen plasmidome was previously reported to contain genes that may confer advantages to their hosts (19). Rat gut (76) and wastewater (149) plasmidomes have also been investigated.
In addition to genes, regulatory sequences in intergenic regions are related to the function of nearby genes. Thus, variations in the comprehensive set of regulatory sequences, or the regulome, for example, by promoter propagation, may play important roles in microbial adaptation (99, 122). We envision direct investigations of regulomes in microbial communities, or a “metaregulome” analysis, becoming an attractive research field as technical advances occur in long-read metagenomics. In a metaregulome analysis, variations and/or possible transfers of regulatory sequences, in addition to those of coding genes, may be identified from metagenomic datasets (Fig. 2B) (48, 122).
Toward a comprehensive analysis of inter-species interactions
Inter-species interactions, including mutualism and parasitism, are of general interest in microbial ecology (16). Using abundant information from large-scale metagenomic datasets, co-occurrences (or anti-occurrences) among microbes, hosts, and/or viruses have been studied, and, for example, species interaction networks have been identified (12, 23, 28, 46, 53, 94, 114, 155, 164, 184). Recent large-scale projects include the Tara Oceans project, which revealed interactions among all three domains and viruses (17). Since environmental samples were revealed to contain environmental DNA shed from large organisms in addition to microbial DNA (107), a combinatorial analysis of microbial and environmental DNA is expected to accelerate the analysis of interactions between microbes and larger organisms.
The viral metagenome is called the metavirome or simply the virome. Viruses also play fundamental roles in ecosystems; therefore, a virome analysis is becoming an important field. To date, viral communities in hypersaline (143, 144) and human gut (105) environments have been extensively studied, and antibiotic viruses have also been of interest (108). A novel bacteriophage present in the majority of published human fecal metagenomes was recently reconstructed (39), and phage-bacteria ecological networks were suggested to protect gut microflora from antibiotic stress (108). Since viruses are classified into different types of DNA and RNA viruses, different approaches must be combined for comprehensive analyses (169). The use of targeted sequence capture techniques to efficiently increase the proportion of viral reads in metagenomic samples may also be considered (179). The largest limitation in bioinformatic analyses of viromes is insufficient reference genome data. Similarity searches using viral sequences often result in no significant hits, suggesting that there are many unknown viruses. In order to overcome this limitation, several bioinformatic tools have been developed and used for virome studies, such as ViromeScan (137) for taxonomy assignment and Metavir 2 (141) for viral genome reconstruction. Another difficulty is that in contrast to prokaryotes that have universal marker genes for a phylogenetic analysis (i.e., 16S rRNA), there is no such gene for viral studies. An analysis of clustered regularly interspaced short palindromic repeats (CRISPRs) is a related emerging field because these repeats represent previous exposures to (or attacks from) viruses (15, 109, 131). CRISPRs are found in approximately 40% of bacteria and approximately 90% of archaea (59), and, thus, a metagenomic analysis of CRISPRs will contribute to advancing the field toward a comprehensive analysis of viral-microbial interactions.
Toward a meta-analysis of metagenomes
Abundant metagenomic datasets containing dozens of terabytes of sequence data are currently found in the Short Read Archive database at NCBI, and its content is increasing daily (84). Whereas each metagenomic dataset provides a snapshot of the microbial community at the time of sampling, a comprehensive analysis (or meta-analysis) of many datasets is expected to reveal general patterns or laws that determine how microbes interact with their environments and how their genomes have been shaped. It is important to note that different datasets have been constructed with different experimental methods and conditions.
Regarding global correlations between environments and microbial genomes, correlations involving genomic GC contents (66) and genome sizes (14) have been reported. MetaMetaDB (181) was developed for a meta-analysis of different environments inhabited by a microbe and the factors that contribute to adaptation. This database may be used to predict all possible habitats of microbes by searching for the presence of microbes in metagenomic and 16S rRNA amplicon sequencing datasets derived from diverse environments. Given a metagenomic or 16S rRNA amplicon sequencing dataset, researchers may find environments with microbial community structures that are similar to that dataset using MetaMetaDB (181). A meta-analysis of metagenomic datasets was also performed to examine microbial adaptation to environments in terms of metabolic flexibility (52, 60) and to investigate specific functional genes that facilitate adaptation to extreme habitats, such as heavy metal resistance genes (65, 106) and salt-stress responsive genes (166). In a meta-analysis, associations were found between membrane protein variations and oceanographic variables in a global ocean sampling expedition (126). Microbial interactions between humans and the indoor environment have also been investigated (91).
Toward metagenomics with long-read sequencers
Sequencers with the ability to produce long-read data are currently being developed, such as the PacBio RS II (Pacific Biosciences, Menlo Park, USA) and nanopore-based sequencers (Oxford Nanopore Technologies, Oxford, UK). Long reads are already contributing to many types of bioinformatic analyses, including the high-quality de novo assembly of bacterial and viral genomes (7, 67) and the detection of genomic structural variations, such as large-scale insertions/deletions or HGTs in microbial communities (71). Long reads are expected to be helpful for reconstructing genomes from metagenome data, directly observing genomic structural variations, and analyzing metaregulomes in various microbial communities. High-density microbial habitats, such as biofilms and gut communities, may be interesting targets because their genomic structures may be changed by the frequent exchange of genetic materials.
Long-read metagenomics will be an emerging field, but there are still limitations to be considered. Although PacBio RS II with P6-C4 chemistry may generate reads with an average length of approximately 15 kb, less than 50,000 reads are generated per SMART cell (i.e., less than 1 Gb in each SMART cell). This throughput is markedly smaller than that of the so-called massively parallel sequencers (e.g., approximately 15 Gb in each run of MiSeq [Illumina]) and may be insufficient for describing taxonomically diverse microbial communities. In addition, the low accuracy of PacBio RS II reads (approximately 85%) may hinder a bioinformatic analysis, unless highly redundant sequencing (e.g., more than 50X coverage) is performed to reach high accuracy in the ensemble. Along with the development of new bioinformatic methods, protocols also need to be optimized to avoid DNA fragmentation during extraction (83, 111).
Concluding remarks
Metagenomic and bioinformatic approaches are already common in microbial ecology and have been used to investigate whole communities containing many types of uncultivable microbes (Fig. 1). However, to date, most analyses have depended on straightforward sequence similarity searches against reference databases. This may not be satisfactory because microbial genomes need to be the fundamental basis for microbial ecology and evolution. The enrichment of reference sequences (for microbial taxa and functional genes) is one of the fundamental issues for promoting various kinds of analyses. Platforms that enable a meta-analysis of diverse metagenomic datasets will allow us to discover the hidden laws of the microbial ecosystem from publicly available data. Long-read sequence information will open up the possibility of studies that focus on subjects that have not yet been examined in detail by using short-read sequences. Furthermore, more powerful bioinformatic methods for analyzing data from diverse perspectives are required in order to advance past routine metagenomic analyses.
Acknowledgements
We thank Tsukasa Fukunaga and Masamichi Takeshita for their helpful suggestions. The ideas and information described in this review were partly developed during research supported by the Japan Science and Technology Agency (CREST), the Japan Society for the Promotion of Science (Grant Numbers 15J08604 and 221S0002), and the Canon Foundation.
References
- 1. Abubucker, S., N. Segata, J. Goll, et al. 2012. Metabolic reconstruction for metagenomic data and its application to the human microbiome. PLoS Comput Biol. 8:e1002358.
- 2. Afiahayati, , K. Sato, and Y. Sakakibara. 2015. MetaVelvet-SL: an extension of the Velvet assembler to a de novo metagenomic assembler utilizing supervised learning. DNA Res. 22:69-77.
- 3. Akkermans, A.D.L., M.S. Mirza, H.J.M. Harmsen, H.J. Blok, P.R. Herron, A. Sessitsch, and W.M. Akkermans. 1994. Molecular ecology of microbes: a review of promises, pitfalls, and true progress. FEMS Microbiol Rev. 15:185-194.
- 4. Albertsen, M., P. Hugenholtz, A. Skarshewski, K.L. Nielsen, G.W. Tyson, and P.H. Nielsen. 2013. Genome sequences of rare, uncultured bacteria obtained by differential coverage binning of multiple metagenomes. Nat Biotechnol. 31:533-538.
- 5. Alneberg, J., B.S. Bjarnason, I. de Bruijn, et al. 2014. Binning metagenomic contigs by coverage and composition. Nat Methods. 11:1144-1146.
- 6. Alonso-Alemany, D., A. Barré, S. Beretta, P. Bonizzoni, M. Nikolski, and G. Valiente. 2014. Further steps in TANGO: improved taxonomic assignment in metagenomics. Bioinformatics. 30:17-23.
- 7. Alquezar-Planas, D.E., T. Mourier, C.A.W. Bruhn, et al. 2013. Discovery of a divergent HPIV4 from respiratory secretions using second and third generation metagenomic sequencing. Sci Rep. 3:2468.
- 8. Banik, J.J., and S.F. Brady. 2008. Cloning and characterization of new glycopeptide gene clusters found in an environmental DNA megalibrary. Proc Natl Acad Sci USA. 105:17273-17277.
- 9. Béjà, O., M.T. Suzuki, E.V. Koonin, et al. 2000. Construction and analysis of bacterial artificial chromosome libraries from a marine microbial assemblage. Environ Microbiol. 2:516-529.
- 10. Belda-Ferre, P., L.D. Alcaraz, R. Cabrera-Rubio, H. Romero, A. Simón-Soro, M. Pignatelli, and A. Mira. 2012. The oral metagenome in health and disease. ISME J. 6:46-56.
- 11. Belton, J.M., R.P. McCord, J.H. Gibcus, N. Naumova, Y. Zhan, and J. Dekker. 2012. Hi-C: a comprehensive technique to capture the conformation of genomes. Methods. 58:268-276.
- 12. Beman, J.M., J.A. Steele, and J.A. Fuhrman. 2011. Co-occurrence patterns for abundant marine archaeal and bacterial lineages in the deep chlorophyll maximum of coastal California. ISME J. 5:1077-1085.
- 13. Bennett, P.M. 2008. Plasmid encoded antibiotic resistance: acquisition and transfer of antibiotic resistance genes in bacteria. Br J Pharmacol. 153:S347-S357.
- 14. Bentkowski, P., C. Van Oosterhout, and T. Mock. 2015. A model of genome size evolution for prokaryotes in stable and fluctuating environments. Genome Biol Evol. 7:2344-2351.
- 15. Bolotin, A., B. Quinquis, A. Sorokin, and S.D. Ehrlich. 2005. Clustered regularly interspaced short palindrome repeats (CRISPRs) have spacers of extrachromosomal origin. Microbiology. 151:2551-2561.
- 16. Boon, E., C.J. Meehan, C. Whidden, D.H.J. Wong, M.G.I. Langille, and R.G. Beiko. 2014. Interactions in the microbiome: communities of organisms and communities of genes. FEMS Microbiol Rev. 38:90-118.
- 17. Bork, P., C. Bowler, C. de Vargas, G. Gorsky, E. Karsenti, and P. Wincker. 2015. Tara Oceans studies plankton at planetary scale. Science. 348:873.
- 18. Brown, C.T., L.A. Hug, B.C. Thomas, et al. 2015. Unusual biology across a group comprising more than 15% of domain Bacteria. Nature. 523:208-211.
- 19. Brown Kav, A., G. Sasson, E. Jami, A. Doron-Faigenboim, I. Benhar, and I. Mizrahi. 2012. Insights into the bovine rumen plasmidome. Proc Natl Acad Sci USA. 109:5452-5457.
- 20. Burton, J.N., I. Liachko, M.J. Dunham, and J. Shendure. 2014. Species-level deconvolution of metagenome assemblies with Hi-C-based contact probability maps. G3. 4:1339-1346.
- 21. Caporaso, J.G., C.L. Lauber, E.K. Costello, et al. 2011. Moving pictures of the human microbiome. Genome Biol. 12:R50.
- 22. Caspi, R., T. Altman, R. Billington, et al. 2014. The MetaCyc database of metabolic pathways and enzymes and the BioCyc collection of Pathway/Genome Databases. Nucleic Acids Res. 42:D459-D471.
- 23. Chaffron, S., H. Rehrauer, J. Pernthaler, and C. Mering. 2010. A global network of coexisting microbes from environmental and whole-genome sequence data. Genome Res. 2010:947-959.
- 24. Chaillou, S., A. Chaulot-Talmon, H. Caekebeke, et al. 2015. Origin and ecological selection of core and food-specific bacterial communities associated with meat and seafood spoilage. ISME J. 9:1105-1118.
- 25. Chen, H.M., and C.H. Lifschitz. 1989. Preparation of fecal samples for assay of volatile fatty acids by gas-liquid chromatography and high-performance liquid chromatography. Clin Chem. 35:74-76.
- 26. Chen, J., and R.P. Novick. 2009. Transfer of toxin genes. Science. 323:139-141.
- 27. Chen, Y., L. Wu, R. Boden, A. Hillebrand, D. Kumaresan, H. Moussard, M. Baciu, Y. Lu, and J.C. Murrell. 2009. Life without light: microbial diversity and evidence of sulfur- and ammonium-based chemolithotrophy in Movile Cave. ISME J. 3:1093-1104.
- 28. Chow, C.-E.T., D.Y. Kim, R. Sachdeva, D.A. Caron, and J.A. Fuhrman. 2014. Top-down controls on bacterial community structure: microbial network analysis of bacteria, T4-like viruses and protists. ISME J. 8:816-829.
- 29. Chun, J., J.-H. Lee, Y. Jung, M. Kim, S. Kim, B.K. Kim, and Y.-W. Lim. 2007. EzTaxon: a web-based tool for the identification of prokaryotes based on 16S ribosomal RNA gene sequences. Int J Syst Evol Microbiol. 57:2259-2261.
- 30. Cleary, B., I.L. Brito, K. Huang, D. Gevers, T. Shea, S. Young, and E.J. Alm. 2015. Detection of low-abundance bacterial strains in metagenomic datasets by eigengenome partitioning. Nat Biotechnol. 33:1053-1060.
- 31. Cole, J.R., Q. Wang, J.A. Fish, et al. 2014. Ribosomal Database Project: data and tools for high throughput rRNA analysis. Nucleic Acids Res. 42:D633-D642.
- 32. Coughlan, L.M., P.D. Cotter, C. Hill, and A. Alvarez-Ordóñez. 2015. Biotechnological applications of functional metagenomics in the food and pharmaceutical industries. Front Microbiol. 6:672.
- 33. Cowan, D.A., J.B. Ramond, T.P. Makhalanyane, and P. De Maayer. 2015. Metagenomics of extreme environments. Curr Opin Microbiol. 25:97-102.
- 34. Culligan, E.P., J.R. Marchesi, C. Hill, and R.D. Sleator. 2012. Mining the human gut microbiome for novel stress resistance genes. Gut Microbes. 3:394-397.
- 35. Darling, A.E., G. Jospin, E. Lowe, F.A. Matsen, H.M. Bik, and J.A. Eisen. 2014. PhyloSift: phylogenetic analysis of genomes and metagenomes. PeerJ. 2:e243.
- 36. Di Bella, J.M., Y. Bao, G.B. Gloor, J.P. Burton, and G. Reid. 2013. High throughput sequencing methods and analysis for microbiome research. J Microbiol Methods. 95:401-414.
- 37. Dib, J.R., M. Wagenknecht, M.E. Farías, and F. Meinhardt. 2015. Strategies and approaches in plasmidome studies-uncovering plasmid diversity disregarding of linear elements? Front Microbiol. 6:463.
- 38. Dostie, J., and J. Dekker. 2007. Mapping networks of physical interactions between genomic elements using 5C technology. Nat Protoc. 2:988-1002.
- 39. Dutilh, B.E., N. Cassman, K. McNair, et al. 2014. A highly abundant bacteriophage discovered in the unknown sequences of human faecal metagenomes. Nat Commun. 5:4498.
- 40. Edgar, R.C. 2010. Search and clustering orders of magnitude faster than BLAST. Bioinformatics. 26:2460-2461.
- 41. Edgar, R.C., B.J. Haas, J.C. Clemente, C. Quince, and R. Knight. 2011. UCHIME improves sensitivity and speed of chimera detection. Bioinformatics. 27:2194-2200.
- 42. Edgar, R.C. 2013. UPARSE: highly accurate OTU sequences from microbial amplicon reads. Nat Methods. 10:996-998.
- 43. Eloe-Fadrosh, E.A., D. Paez-Espino, J. Jarett, et al. 2016. Global metagenomic survey reveals a new bacterial candidate phylum in geothermal springs. Nat Commun. 7:10476.
- 44. Erickson, A.R., B.L. Cantarel, R. Lamendella, et al. 2012. Integrated metagenomics/metaproteomics reveals human host-microbiota signatures of Crohn’s disease. PLoS One. 7:e49138.
- 45. Evans, P.N., D.H. Parks, G.L. Chadwick, S.J. Robbins, V.J. Orphan, S.D. Golding, and G.W. Tyson. 2015. Methane metabolism in the archaeal phylum Bathyarchaeota revealed by genome-centric metagenomics. Science. 350:434-438.
- 46. Faust, K., and J. Raes. 2012. Microbial interactions: from networks to models. Nat Rev Microbiol. 10:538-550.
- 47. Felczykowska, A., S.K. Bloch, B. Nejman-Faleńczyk, and S. Barańska. 2012. Metagenomic approach in the investigation of new bioactive compounds in the marine environment. Acta Biochim Pol. 59:501-505.
- 48. Fernandez, L., J.M. Mercader, M. Planas-Fèlix, and D. Torrents. 2014. Adaptation to environmental factors shapes the organization of regulatory regions in microbial communities. BMC Genomics. 15:877.
- 49. Fierer, N., C.C.L. Lauber, N. Zhou, D. McDonald, E.K. Costello, and R. Knight. 2010. Forensic identification using skin bacterial communities. Proc Natl Acad Sci USA. 107:6477-6481.
- 50. Fu, L., B. Niu, Z. Zhu, S. Wu, and W. Li. 2012. CD-HIT: accelerated for clustering the next-generation sequencing data. Bioinformatics. 28:3150-3152.
- 51. Gatte-Picchi, D., A. Weiz, K. Ishida, C. Hertweck, and E. Dittmann. 2014. Functional analysis of environmental DNA-derived microviridins provides new insights into the diversity of the tricyclic peptide family. Appl Environ Microbiol. 80:1380-1387.
- 52. Gianoulis, T.A., J. Raes, P.V. Patel, et al. 2009. Quantifying environmental adaptation of metabolic pathways in metagenomics. Proc Natl Acad Sci USA. 106:1374-1379.
- 53. Gilbert, J.A. 2012. Defining seasonal marine microbial community dynamics. ISME J. 6:298-308.
- 54. Gilbert, J.A., J.K. Jansson, and R. Knight. 2014. The Earth Microbiome project: successes and aspirations. BMC Biol. 12:69.
- 55. Gill, S.R., M. Pop, R.T. Deboy, et al. 2006. Metagenomic analysis of the human distal gut microbiome. Science. 312:1355-1359.
- 56. Gillespie, D.E., S.F. Brady, A.D. Bettermann, N.P. Cianciotto, M.R. Liles, M.R. Rondon, J. Clardy, R.M. Goodman, and J. Handelsman. 2002. Isolation of antibiotics turbomycin A and B from a metagenomic library of soil microbial DNA. Appl Environ Microbiol. 68:4301-4306.
- 57. Greenblum, S., P.J. Turnbaugh, and E. Borenstein. 2012. Metagenomic systems biology of the human gut microbiome reveals topological shifts associated with obesity and inflammatory bowel disease. Proc Natl Acad Sci USA. 109:594-599.
- 58. Grigoriev, I.V., R. Nikitin, S. Haridas, et al. 2014. MycoCosm portal: gearing up for 1000 fungal genomes. Nucleic Acids Res. 42:D699-D704.
- 59. Grissa, I., G. Vergnaud, and C. Pourcel. 2007. The CRISPRdb database and tools to display CRISPRs and to generate dictionaries of spacers and repeats. BMC Bioinformatics. 8:172.
- 60. Grzymski, J.J., A.E. Murray, B.J. Campbell, et al. 2008. Metagenome analysis of an extreme microbial symbiosis reveals eurythermal adaptation and metabolic flexibility. Proc Natl Acad Sci USA. 105:17516-17521.
- 61. Guazzaroni, M.E., V. Morgante, S. Mirete, and J.E. González-Pastor. 2013. Novel acid resistance genes from the metagenome of the Tinto River, an extremely acidic environment. Environ Microbiol. 15:1088-1102.
- 62. Guo, J., Q. Wang, X. Wang, F. Wang, J. Yao, and H. Zhu. 2015. Horizontal gene transfer in an acid mine drainage microbial community. BMC Genomics. 16:496.
- 63. Haas, B.J., D. Gevers, A.M. Earl, et al. 2011. Chimeric 16S rRNA sequence formation and detection in Sanger and 454-pyrosequenced PCR amplicons. Genome Res. 21:494-504.
- 64. Hastie, A.R., L. Dong, A. Smith, et al. 2013. Rapid genome mapping in nanochannel arrays for highly complete and accurate de novo sequence assembly of the complex Aegilops tauschii Genome. PLoS One. 8:e55864.
- 65. Hemme, C.L., Y. Deng, T.J. Gentry, et al. 2010. Metagenomic insights into evolution of a heavy metal-contaminated groundwater microbial community. ISME J. 4:660-672.
- 66. Hildebrand, F., A. Meyer, and A. Eyre-walker. 2010. Evidence of selection on genomic GC content in bacteria. PLoS Genet. 6:e1001107.
- 67. Hiraoka, S., A. Machiyama, M. Ijichi, K. Inoue, K. Oshima, M. Hattori, S. Yoshizawa, K. Kogure, and W. Iwasaki. 2016. Genomic and metagenomic analysis of microbes in a soil environment affected by the 2011 Great East Japan Earthquake tsunami. BMC Genomics. 17:53.
- 68. Hongoh, Y. 2010. Diversity and genomes of uncultured microbial symbionts in the termite gut. Biosci Biotechnol Biochem. 74:1145-1151.
- 69. Hunter, S., M. Corbett, H. Denise, et al. 2014. EBI metagenomics—a new resource for the analysis and archiving of metagenomic data. Nucleic Acids Res. 42:D600-D606.
- 70. Huson, D.H., and N. Weber. 2013. Microbial community analysis using MEGAN. Methods Enzymol. 531:465-485.
- 71. Ikuta, T., Y. Takaki, Y. Nagai, et al. 2016. Heterogeneous composition of key metabolic gene clusters in a vent mussel symbiont population. ISME J. 10:990-1001.
- 72. Iwasaki, W., and T. Takagi. 2007. Reconstruction of highly heterogeneous gene-content evolution across the three domains of life. Bioinformatics. 23:i230-i239.
- 73. Iwasaki, W., and T. Takagi. 2009. Rapid pathway evolution facilitated by horizontal gene transfers across prokaryotic lineages. PLoS Genet. 5:e1000402.
- 74. Jackson, S.A., E. Borchert, F. O’Gara, and A.D.W. Dobson. 2015. Metagenomics for the discovery of novel biosurfactants of environmental interest from marine ecosystems. Curr Opin Biotechnol. 33:176-182.
- 75. Jiao, D., Y. Ye, and H. Tang. 2013. Probabilistic inference of biochemical reactions in microbial communities from metagenomic sequences. PLoS Comput Biol. 9:e1002981.
- 76. Jørgensen, T.S., Z. Xu, M.A. Hansen, S.J. Sørensen, and L.H. Hansen. 2014. Hundreds of circular novel plasmids and DNA elements identified in a rat cecum metamobilome. PLoS One. 9:e87924.
- 77. Jung, J.Y., S.H. Lee, J.M. Kim, M.S. Park, J.W. Bae, Y. Hahn, E.L. Madsen, and C.O. Jeon. 2011. Metagenomic analysis of kimchi, a Traditional Korean fermented food. Appl Environ Microbiol. 77:2264-2274.
- 78. Kagan, J., I. Sharon, O. Beja, and J.C. Kuhn. 2008. The tryptophan pathway genes of the Sargasso Sea metagenome: new operon structures and the prevalence of non-operon organization. Genome Biol. 9:R20.
- 79. Kakizaki, E., Y. Ogura, S. Kozawa, S. Nishida, T. Uchiyama, T. Hayashi, and N. Yukawa. 2012. Detection of diverse aquatic microbes in blood and organs of drowning victims: first metagenomic approach using high-throughput 454-pyrosequencing. Forensic Sci Int. 220:135-146.
- 80. Kanehisa, M., S. Goto, Y. Sato, M. Furumichi, and M. Tanabe. 2012. KEGG for integration and interpretation of large-scale molecular data sets. Nucleic Acids Res. 40:D109-D114.
- 81. Kang, D.D., J. Froula, R. Egan, and Z. Wang. 2015. MetaBAT, an efficient tool for accurately reconstructing single genomes from complex microbial communities. PeerJ. 3:e1165.
- 82. Khodakova, A.S., R.J. Smith, L. Burgoyne, D. Abarno, and A. Linacre. 2014. Random whole metagenomic sequencing for forensic discrimination of soils. PLoS One. 9:e104996.
- 83. Kim, S.W., W. Suda, S. Kim, K. Oshima, S. Fukuda, H. Ohno, H. Morita, and M. Hattori. 2013. Robustness of gut microbiota of healthy adults in response to probiotic intervention revealed by high-throughput pyrosequencing. DNA Res. 20:241-253.
- 84. Kodama, Y., M. Shumway, and R. Leinonen. 2012. The sequence read archive: explosive growth of sequencing data. Nucleic Acids Res. 40:D54-D56.
- 85. Kong, H.H., J. Oh, C. Deming, et al. 2012. Temporal shifts in the skin microbiome associated with disease flares and treatment in children with atopic dermatitis. Genome Res. 22:850-859.
- 86. Korem, T., D. Zeevi, J. Suez, et al. 2015. Growth dynamics of gut microbiota in health and disease inferred from single metagenomic samples. Science. 349:1101-1106.
- 87. Kostic, A.D., D. Gevers, C.S. Pedamallu, et al. 2012. Genomic analysis identifies association of Fusobacterium with colorectal carcinoma. Genome Res. 22:292-298.
- 88. Kunin, V., A. Copeland, A. Lapidus, K. Mavromatis, and P. Hugenholtz. 2008. A bioinformatician’s guide to metagenomics. Microbiol Mol Biol Rev. 72:557-578.
- 89. Langille, M.G., J. Zaneveld, J.G. Caporaso, et al. 2013. Predictive functional profiling of microbial communities using 16S rRNA marker gene sequences. Nat Biotechnol. 31:814-821.
- 90. Lasken, R.S. 2012. Genomic sequencing of uncultured microorganisms from single cells. Nat Rev Microbiol. 10:631-640.
- 91. Lax, S., D.P. Smith, J. Hampton-Marcell, et al. 2014. Longitudinal analysis of microbial interaction between humans and the indoor environment. Science. 345:1048-1052.
- 92. Lewin, A., A. Wentzel, and S. Valla. 2013. Metagenomics of microbial life in extreme temperature environments. Curr Opin Biotechnol. 24:516-525.
- 93. Li, R.W. 2011. Metagenomics and Its Applications in Agriculture, Biomedicine, and Environmental Studies. Nova Science Publisher’s.
- 94. Lozupone, C., K. Faust, J. Raes, J.J. Faith, D.N. Frank, J. Zaneveld, J.I. Gordon, and R. Knight. 2012. Identifying genomic and metabolic features that can underlie early successional and opportunistic lifestyles of human gut symbionts. Genome Res. 22:1974-1984.
- 95. Luo, C., D. Tsementzi, N.C. Kyrpides, and K.T. Konstantinidis. 2012. Individual genome assembly from complex community short-read metagenomic datasets. ISME J. 6:898-901.
- 96. Manichanh, C., L. Rigottier-Gois, E. Bonnaud, et al. 2006. Reduced diversity of faecal microbiota in Crohn’s disease revealed by a metagenomic approach. Gut. 55:205-211.
- 97. Markowitz, V.M., I.M.A. Chen, K. Chu, et al. 2014. IMG/M 4 version of the integrated metagenome comparative analysis system. Nucleic Acids Res. 42:D568-D573.
- 98. Matsen, F.A., R.B. Kodner, and E.V. Armbrust. 2010. pplacer: linear time maximum-likelihood and Bayesian phylogenetic placement of sequences onto a fixed reference tree. BMC Bioinformatics. 11:538.
- 99. Matus-Garcia, M., H. Nijveen, and M.W.J. Van Passel. 2012. Promoter propagation in prokaryotes. Nucleic Acids Res. 40:10032-10040.
- 100. Mavromatis, K., N. Ivanova, K.W. Barry, et al. 2007. Use of simulated data sets to evaluate the fidelity of metagenomic processing methods. Nat Methods. 4:495-500.
- 101. McDonald, D., M.N. Price, J. Goodrich, E.P. Nawrocki, T.Z. DeSantis, A. Probst, G.L. Andersen, R. Knight, and P. Hugenholtz. 2012. An improved Greengenes taxonomy with explicit ranks for ecological and evolutionary analyses of bacteria and archaea. ISME J. 6:610-618.
- 102. Mende, D.R., F.O. Aylward, J.M. Eppley, T.N. Nielsen, and E.F. DeLong. 2016. Improved environmental genomes via integration of metagenomic and single-cell assemblies. Front Microbiol. 7:143.
- 103. Mendoza, M.L.Z., T. Sicheritz-Pontén, and M.P.T. Gilbert. 2014. Environmental genes and genomes: understanding the differences and challenges in the approaches and software for their analyses. Brief Bioinform. 16:745-758.
- 104. Meyer, F., D. Paarmann, M. D’Souza, et al. 2008. The metagenomics RAST server—a public resource for the automatic phylogenetic and functional analysis of metagenomes. BMC Bioinformatics. 9:386.
- 105. Minot, S., R. Sinha, J. Chen, H. Li, S.A. Keilbaugh, G.D. Wu, J.D. Lewis, and F.D. Bushman. 2011. The human gut virome: inter-individual variation and dynamic response to diet. Genome Res. 21:1616-1625.
- 106. Mirete, S., C.G. De Figueras, and J.E. González-Pastor. 2007. Novel nickel resistance genes from the rhizosphere metagenome of plants adapted to acid mine drainage. Appl Environ Microbiol. 73:6001-6011.
- 107. Miya, M., Y. Sato, T. Fukunaga, et al. 2015. MiFish, a set of universal PCR primers for metabarcoding environmental DNA from fishes: detection of more than 230 subtropical marine species. R Soc Open Sci. 2:150088.
- 108. Modi, S.R., H.H. Lee, C.S. Spina, and J.J. Collins. 2013. Antibiotic treatment expands the resistance reservoir and ecological network of the phage metagenome. Nature. 499:219-222.
- 109. Mojica, F.J.M., C. Díez-Villaseñor, J. García-Martínez, and E. Soria. 2005. Intervening sequences of regularly spaced prokaryotic repeats derive from foreign genetic elements. J Mol Evol. 60:174-182.
- 110. Mori, K., and Y. Kamagata. 2014. The Challenges of studying the anaerobic microbial world. Microbes Environ. 29:335-337.
- 111. Morita, H., T. Kuwahara, K. Ohshima, H. Sasamoto, K. Itoh, M. Hattori, T. Hayashi, and H. Takami. 2007. An improved DNA isolation method for metagenomic analysis of the microbial flora of the human intestine. Microbes Environ. 22:214-222.
- 112. Narasingarao, P., S. Podell, J.A. Ugalde, C. Brochier-Armanet, J.B. Emerson, J.J. Brocks, K.B. Heidelberg, J.F. Banfield, and E.E. Allen. 2012. De novo metagenomic assembly reveals abundant novel major lineage of Archaea in hypersaline microbial communities. ISME J. 6:81-93.
- 113. Narihiro, T., and Y. Kamagata. 2013. Cultivating yet-to-be cultivated microbes: the challenge continues. Microbes Environ. 28:163-165.
- 114. Navarrete, A.A., S.M. Tsai, L.W. Mendes, K. Faust, M. De Hollander, N.A. Cassman, J. Raes, J.A. Van Veen, and E.E. Kuramae. 2015. Soil microbiome responses to the short-term effects of Amazonian deforestation. Mol Ecol. 24:2433-2448.
- 115. Neelakanta, G., and H. Sultana. 2013. The use of metagenomic approaches to analyze changes in microbial communities. Microbiol Insights. 6:37-48.
- 116. Nelson, K.E., J.L. Peterson, and S. Garges. 2011. Metagenomics of the human body, p.1-351. In K.E. Nelson (ed.), Metagenomics of the Human Body. Springer.
- 117. Nielsen, H.B., M. Almeida, A.S. Juncker, et al. 2014. Identification and assembly of genomes and genetic elements in complex metagenomic samples without using reference genomes. Nat Biotechnol. 32:822-828.
- 118. Nijkamp, J.F., M. Pop, M.J.T. Reinders, and D. De Ridder. 2013. Exploring variation-aware contig graphs for (comparative) metagenomics using MARYGOLD. Bioinformatics. 29:2826-2834.
- 119. Nurk, S., A. Bankevich, D. Antipov, et al. 2013. Assembling single-cell genomes and mini-metagenomes from chimeric MDA products. J Comput Biol. 20:714-737.
- 120. Ochman, H., J.G. Lawrence, and E.A. Groisman. 2000. Lateral gene transfer and the nature of bacterial innovation. Nature. 405:299-304.
- 121. Okuda, S., Y. Tsuchiya, C. Kiriyama, M. Itoh, and H. Morisaki. 2012. Virtual metagenome reconstruction from 16S rRNA gene sequences. Nat Commun. 3:1203.
- 122. Oren, Y., M.B. Smith, N.I. Johns, et al. 2014. Transfer of noncoding DNA drives regulatory rewiring in bacteria. Proc Natl Acad Sci USA. 111:16112-16117.
- 123. Oulas, A., C. Pavloudi, P. Polymenakou, G.A. Pavlopoulos, N. Papanikolaou, G. Kotoulas, C. Arvanitidis, and I. Iliopoulos. 2015. Metagenomics: tools and insights for analyzing next-generation sequencing data derived from biodiversity studies. Bioinform Biol Insights. 9:75-88.
- 124. Overbeek, R., T. Begley, R.M. Butler, et al. 2005. The subsystems approach to genome annotation and its use in the project to annotate 1000 genomes. Nucleic Acids Res. 33:5691-5702.
- 125. Palenik, B., Q. Ren, V. Tai, and I.T. Paulsen. 2009. Coastal Synechococcus metagenome reveals major roles for horizontal gene transfer and plasmids in population diversity. Environ Microbiol. 11:349-359.
- 126. Patel, P.V., T.A. Gianoulis, R.D. Bjornson, K.Y. Yip, D.M. Engelman, and M.B. Gerstein. 2010. Analysis of membrane proteins in metagenomics: networks of correlated environmental features and protein families. Genome Res. 20:960-971.
- 127. Peng, Y., H.C.M. Leung, S.M. Yiu, and F.Y.L. Chin. 2012. IDBA-UD: A de novo assembler for single-cell and metagenomic sequencing data with highly uneven depth. Bioinformatics. 28:1420-1428.
- 128. Pignatelli, M., G. Aparicio, I. Blanquer, V. Hernández, A. Moya, and J. Tamames. 2008. Metagenomics reveals our incomplete knowledge of global diversity. Bioinformatics. 24:2124-2125.
- 129. Pignatelli, M., and A. Moya. 2011. Evaluating the fidelity of de novo short read metagenomic assembly using simulated data. PLoS One. 6:e19984.
- 130. Pop, M., A. Phillippy, and A.L. Delcher. 2004. Comparative genome assembly. Bioinformatics. 5:237-248.
- 131. Pourcel, C., G. Salvignol, and G. Vergnaud. 2005. CRISPR elements in Yersinia pestis acquire new repeats by preferential uptake of bacteriophage DNA, and provide additional tools for evolutionary studies. Microbiology. 151:653-663.
- 132. Prosser, J.I. 2015. Dispersing misconceptions and identifying opportunities for the use of “omics” in soil microbial ecology. Nat Rev Microbiol. 13:439.
- 133. Puspita, I.D., Y. Kamagata, M. Tanaka, K. Asano, and C.H. Nakatsu. 2012. Are uncultivated bacteria really uncultivable? Microbes Environ. 27:356-366.
- 134. Qin, J., Y. Li, Z. Cai, et al. 2012. A metagenome-wide association study of gut microbiota in type 2 diabetes. Nature. 490:55-60.
- 135. Quast, C., E. Pruesse, P. Yilmaz, J. Gerken, T. Schweer, P. Yarza, J. Peplies, and F.O. Glöckner. 2013. The SILVA ribosomal RNA gene database project: improved data processing and web-based tools. Nucleic Acids Res. 41:D590-D596.
- 136. Quince, C., A. Lanzen, R.J. Davenport, and P.J. Turnbaugh. 2011. Removing noise from pyrosequenced amplicons. BMC Bioinformatics. 12:38.
- 137. Rampelli, S., M. Soverini, S. Turroni, S. Quercia, E. Biagi, P. Brigidi, and M. Candela. 2016. ViromeScan: a new tool for metagenomic viral community profiling. BMC Genomics. 17:165.
- 138. Rappé, M.S., and S.J. Giovannoni. 2003. The uncultured microbial majority. Annu Rev Microbiol. 57:369-394.
- 139. Roberts, A.P., and J. Kreth. 2014. The impact of horizontal gene transfer on the adaptive ability of the human oral microbiome. Front Cell Infect Microbiol. 4:124.
- 140. Rodrigue, S., R.R. Malmstrom, A.M. Berlin, B.W. Birren, M.R. Henn, and S.W. Chisholm. 2009. Whole genome amplification and de novo assembly of single bacterial cells. PLoS One. 4:e6864.
- 141. Roux, S., J. Tournayre, A. Mahul, D. Debroas, and F. Enault. 2014. Metavir 2: new tools for viral metagenome comparison and assembled virome analysis. BMC Bioinformatics. 15:76.
- 142. Rusch, D.B., A.L. Halpern, G. Sutton, et al. 2007. The sorcerer II global ocean sampling expedition: northwest atlantic through eastern tropical pacific. PLoS Biol. 5:0398-0431.
- 143. Santos, F., P. Yarza, V. Parro, C. Briones, and J. Antón. 2010. The metavirome of a hypersaline environment. Environ Microbiol. 12:2965-2976.
- 144. Santos, F., P. Yarza, V. Parro, I. Meseguer, R. Rosselló-Móra, and J. Antón. 2012. Culture-independent approaches for studying viruses from hypersaline environments. Appl Environ Microbiol. 78:1635-1643.
- 145. Schloss, P.D., S.L. Westcott, T. Ryabin, et al. 2009. Introducing mothur: open-source, platform-independent, community-supported software for describing and comparing microbial communities. Appl Environ Microbiol. 75:7537-7541.
- 146. Scholz, M.B., C.C. Lo, and P.S.G. Chain. 2012. Next generation sequencing and bioinformatic bottlenecks: the current state of metagenomic data analysis. Curr Opin Biotechnol. 23:9-15.
- 147. Schwabe, R.F., and C. Jobin. 2013. The microbiome and cancer. Nat Rev Cancer. 13:800-812.
- 148. Segata, N., L. Waldron, A. Ballarini, V. Narasimhan, O. Jousson, and C. Huttenhower. 2012. Metagenomic microbial community profiling using unique clade-specific marker genes. Nat Methods. 9:811-814.
- 149. Sentchilo, V., A.P. Mayer, L. Guy, et al. 2013. Community-wide plasmid gene mobilization and selection. ISME J. 7:1173-1186.
- 150. Sharpton, T.J., S.J. Riesenfeld, S.W. Kembel, J. Ladau, J.P. O’Dwyer, J.L. Green, J.A. Eisen, and K.S. Pollard. 2011. PhyLOTU: a high-throughput procedure quantifies microbial community diversity and resolves novel taxa from metagenomic data. PLoS Comput Biol. 7:e1001061.
- 151. Silver, S., and T.K. Misra. 1988. Plasmid-mediated heavy metal resistances. Annu Rev Microbiol. 42:717-743.
- 152. Smalla, K., and P.A. Sobecky. 2002. The prevalence and diversity of mobile genetic elements in bacterial communities of different environmental habitats: insights gained from different methodological approaches. FEMS Microbiol Ecol. 42:165-175.
- 153. Smillie, C.S., M.B. Smith, J. Friedman, O.X. Cordero, L.A. David, and E.J. Alm. 2011. Ecology drives a global network of gene exchange connecting the human microbiome. Nature. 480:241-244.
- 154. Snel, B., P. Bork, and M.A. Huynen. 2002. Genomes in flux: the evolution of Archaeal and Proteobacterial gene content. Genome Res. 12:17-25.
- 155. Soffer, N., J. Zaneveld, and R.V. Thurber. 2015. Phage-bacteria network analysis and its implication for the understanding of coral disease. Environ Microbiol. 17:1203-1218.
- 156. Soucy, S.M., J. Huang, and J.P. Gogarten. 2015. Horizontal gene transfer: building the web of life. Nat Rev Genet. 16:472-482.
- 157. Su, C., L. Lei, Y. Duan, K.Q. Zhang, and J. Yang. 2012. Culture-independent methods for studying environmental microorganisms: methods, application, and perspective. Appl Microbiol Biotechnol. 93:993-1003.
- 158. Suenaga, H., Y. Koyama, M. Miyakoshi, R. Miyazaki, H. Yano, M. Sota, Y. Ohtsubo, M. Tsuda, and K. Miyazaki. 2009. Novel organization of aromatic degradation pathway genes in a microbial community as revealed by metagenomic analysis. ISME J. 3:1335-1348.
- 159. Sunagawa, S., D.R. Mende, G. Zeller, et al. 2013. Metagenomic species profiling using universal phylogenetic marker genes. Nat Methods. 10:1196-1199.
- 160. Takami, H., T. Taniguchi, Y. Moriya, T. Kuwahara, M. Kanehisa, and S. Goto. 2012. Evaluation method for the potential functionome harbored in the genome and metagenome. BMC Genomics. 13:699.
- 161. Tamames, J., and A. Moya. 2008. Estimating the extent of horizontal gene transfer in metagenomic sequences. BMC Genomics. 9:136.
- 162. Tasse, L., J. Bercovici, S. Pizzut-Serin, et al. 2010. Functional metagenomics to mine the human gut microbiome for dietary fiber catabolic enzymes. Genome Res. 20:1605-1612.
- 163. Tatusova, T., S. Ciufo, B. Fedorov, K. O’Neill, and I. Tolstoy. 2014. RefSeq microbial genomes database: new representation and annotation strategy. Nucleic Acids Res. 42:D553-D559.
- 164. Toju, H., P.R. Guimarães, J.M. Olesen, and J.N. Thompson. 2014. Assembly of complex plant–fungus networks. Nat Commun. 5:5273.
- 165. Top, E.M., W.E. Holben, and L.J. Forney. 1995. Characterization of diverse 2,4-dichlorophenoxyacetic acid-degradative plasmids isolated from soil by complementation. Appl Environ Microbiol. 61:1691-1698.
- 166. Trabelsi, D., A. Mengoni, M.E. Aouani, M. Bazzicalupo, and R. Mhamdi. 2010. Genetic diversity and salt tolerance of sinorhizobium populations from two tunisian soils. Ann Microbiol. 60:541-547.
- 167. Turnbaugh, P.J., R.E. Ley, M. Hamady, C.M. Fraser-Liggett, R. Knight, and J.I. Gordon. 2007. Feature the human microbiome project. Nature. 449:804-810.
- 168. Tyson, G.W., J. Chapman, P. Hugenholtz, et al. 2004. Community structure and metabolism through reconstruction of microbial genomes from the environment. Nature. 428:37-43.
- 169. Urayama, S., Y. Yoshida-Takashima, M. Yoshida, Y. Tomaru, H. Moriyama, K. Takai, and T. Nunoura. 2015. A new fractionation and recovery method of viral genomes based on nucleic acid composition and structure using tandem column chromatography. Microbes Environ. 30:199-203.
- 170. Vázquez-Castellanos, J.F., R. García-López, V. Pérez-Brocal, M. Pignatelli, and A. Moya. 2014. Comparison of different assembly and annotation tools on analysis of simulated viral metagenomic communities in the gut. BMC Genomics. 15:37.
- 171. Vogel, T.M., P. Simonet, J.K. Jansson, et al. 2009. TerraGenome: a consortium for the sequencing of a soil metagenome. Nat Rev Microbiol. 7:252.
- 172. Voigt, A.Y., P.I. Costea, J.R. Kultima, S.S. Li, G. Zeller, S. Sunagawa, and P. Bork. 2015. Temporal and technical variability of human gut metagenomes. Genome Biol. 16:73.
- 173. Walker, A. 2012. Welcome to the plasmidome. Nat Rev Microbiol. 467:379.
- 174. Warnecke, F., P. Luginbühl, N. Ivanova, et al. 2007. Metagenomic and functional analysis of hindgut microbiota of a wood-feeding higher termite. Nature. 450:560-565.
- 175. Wichmann, F., N. Udikovic-Kolic, S. Andrew, and J. Handelsman. 2014. Diverse antibiotic resistance genes in dairy cow manure. MBio. 5:e01017-13.
- 176. Wood, D.E., and S.L. Salzberg. 2014. Kraken: ultrafast metagenomic sequence classification using exact alignments. Genome Biol. 15:R46.
- 177. Woyke, T., H. Teeling, N.N. Ivanova, et al. 2006. Symbiosis insights through metagenomic analysis of a microbial consortium. Nature. 443:950-955.
- 178. Wu, Y.-W., Y.-H. Tang, S.G. Tringe, B.A. Simmons, and S.W. Singer. 2014. MaxBin: an automated binning method to recover individual genomes from metagenomes using an expectation-maximization algorithm. Microbiome. 2:26.
- 179. Wylie, T.N., K.M. Wylie, B.N. Herter, G.A. Storch, and T.N. Wylie. 2015. Enhanced virome sequencing using targeted sequence capture. Genome. 25:1910-1920.
- 180. Yamazaki, Y., R. Akashi, Y. Banno, et al. 2010. NBRP databases: databases of biological resources in Japan. Nucleic Acids Res. 38:D26-D32.
- 181. Yang, C.C., and W. Iwasaki. 2014. MetaMetaDB: a database and analytic system for investigating microbial habitability. PLoS One. 9:e87126.
- 182. Yang, Y., B. Xie, and J. Yan. 2014. Application of next-generation sequencing technology in forensic science. Genomics Proteomics Bioinformatics. 12:190-197.
- 183. Ye, Y., and T.G. Doak. 2011. A parsimony approach to biological pathway reconstruction/inference for metagenomes. PLoS Comput Biol. 5:e1000465.
- 184. Zhang, Y., C.Y. Lun, and S.K.W. Tsui. 2015. Metagenomics: a newway to illustrate the crosstalk between infectious diseases and host microbiome. Int J Mol Sci. 16:26263-26279.
- 185. Zoetendal, E.G., E.E. Vaughan, and W.M. De Vos. 2006. A microbial world within us. Mol Microbiol. 59:1639-1650.