In this study, we used a benchmark dataset to evaluate the impact of scaling with the extrapolation domain on the prediction performance of machine learning algorithms. We pseudo-divided the data into the interpolation domain (training data) and the extrapolation domain (test data) using a combination of UMAP (Uniform Manifold Approximation and Projection) and material domain knowledge. In anticipation of bridging interpolation and extrapolation domains in nonlinear machine learning algorithms, we evaluated how the scaling considering the extrapolation domain affects prediction performance in the extrapolation domain. For this evaluation, we used three nonlinear algorithms widely used in the MI (Materials Informatics) domain: XGB (XGBoost) regression, GP (Gaussian Process) regression, and SVR (Support Vector Regression). In this study, by defining the pseudo extrapolation domain, we established the approach for evaluating the prediction accuracy of machine learning models in the extrapolation domain, which is considered difficult to evaluate quantitatively. We also demonstrated that this method, which uses scaling that considers the extrapolation domain, is an effective method for improving prediction accuracy in the extrapolation domain while maintaining prediction accuracy in the interpolation domain.
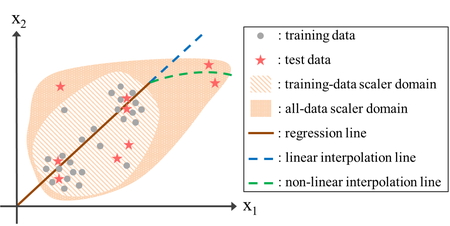
View full abstract